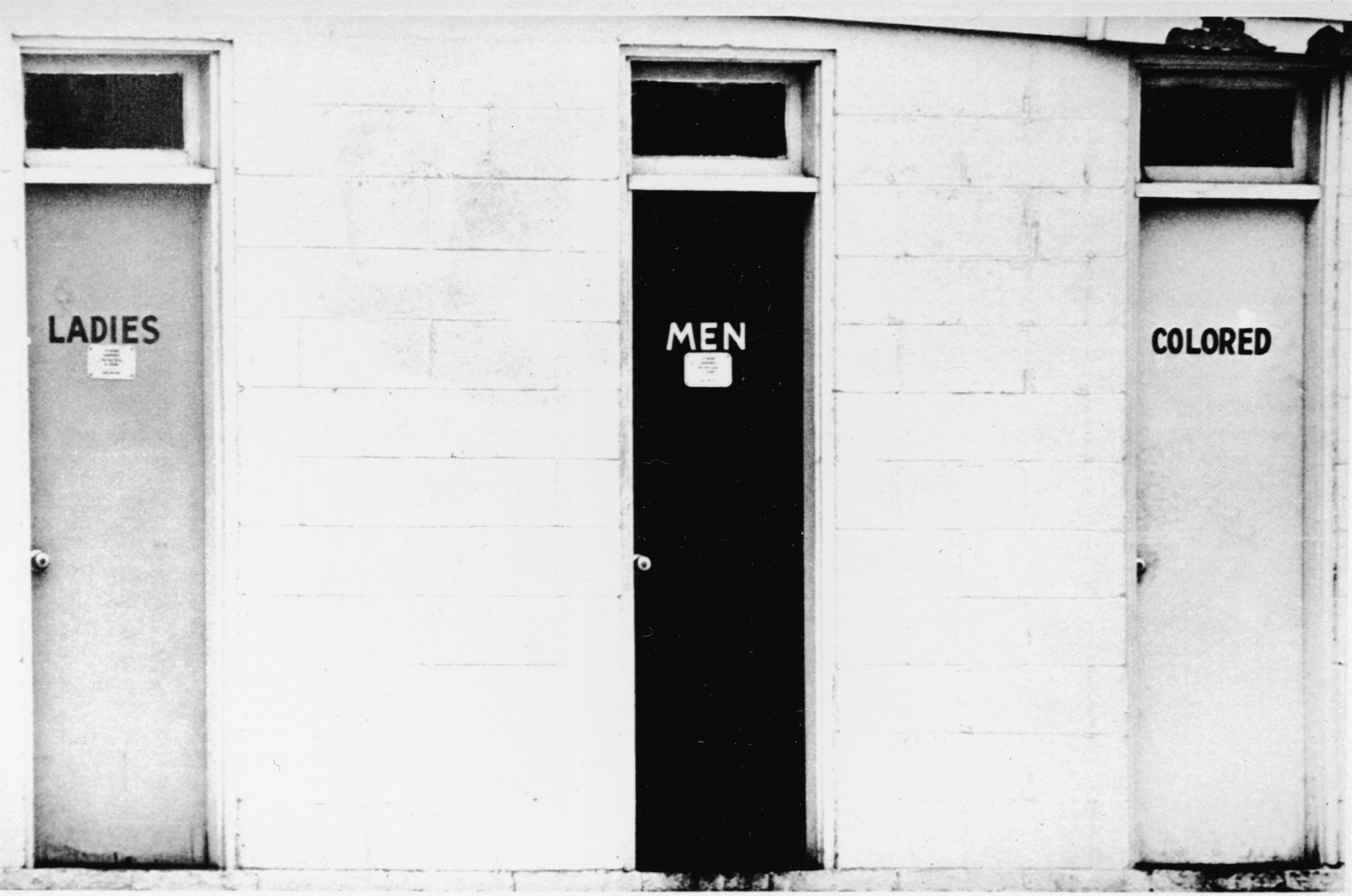
If you’re using the loo in a public place, which side up do you put your hands underneath the soap dispenser? The lighter your skin, the less likely you are to automatically know the answer: or even to understand the question.
But the darker your skin, the more likely it is that you’ll know that the only way to get it to work is to make sure that the palms of your hands, which are lighter, are facing upwards at the sensor.
That’s not because the soap dispensing industry is filled with diehard segregationists who don’t want dark-skinned people in communal bathrooms, but because the hardest thing about designing a soap dispenser – or indeed, any automated sensor – is making sure that it doesn’t spray soap over every passing moth, the corner of someone’s handbag, or anything other than a human hand. Yet because the people given the task of making the dispenser – of actually designing and testing it – tend to have lighter skin, this problem, more often than not, is only spotted when the finished product is out and being used in the real world.
That’s the simplest example of a “racist algorithm”: a series of assumptions made by a machine or system that, when taken together, result in the machine or system discriminating on the grounds of race. But, of course, the problem – which congresswoman Alexandria Ocasio-Cortez got flak for discussing at an event on Monday – is more widespread and has more serious consequences than me having to think about which way up my hands need to be to get the soap out of a dispenser in a public toilet.
An algorithm is essentially just a series of assumptions with a result at the end: it takes in information, runs it against a set of criteria, and arrives at an answer. For example, if you wanted to design an algorithm to decide whether or not to invite someone to your wedding, you might design something to exclude anyone you hadn’t spoken to in the past year; of that list, you might then want to prune it to exclude anyone from your place of work or who hadn’t invited you to their wedding.
But any algorithm is only as a good as the assumptions that are fed into it, and if you aren’t careful, you can end up with troubling results.
In the United Kingdom, algorithms are already used in public life, with mixed results. The County Durham police force uses a system called “Hart”, the Harm Assessment Risk Tool, to assess whether or not people in custody are likely to reoffend in order to better target resources to help prevent people from slipping back into criminal behaviour. The Department of Work and Pensions uses algorithms to assess whether or not welfare claims are fraudulent, flagging potentially fraudulent cases to a human investigator. And the Metropolitan Police uses facial recognition technology to scan its “watch-list” for potential offenders in crowds.
But even across those three uses, the outcomes have been wildly different. Hart is vastly superior to the system of human intuition that preceded it: it correctly categorises the risk 90 per cent of the time. The development and testing of Hart has also been a case study in how to deploy algorithms in public policy: its assumptions and inputs are freely available and its deployment has been the subject of considerable testing.
But the DWP, one of the least transparent departments, is a case study in how not to deploy algorithms, because it is impossible to tell how effectively they are being used. The Metropolitan Police’s use of facial identification algorithms has been conducted with more transparency than the DWP’s use of algorithms has, but it has also been a failure, initially producing incorrect identification in 98 per cent of cases when trialled. (It has improved somewhat since.)
We don’t yet know if any of the underlying assumptions used in Hart discriminate on the grounds of race or gender yet, because it has not been deployed for policing in a diverse area, and the proportion of female offenders is so low that it is hard to draw statistically significant conclusions yet. (We would expect any discrimination in algorithmic policing to hit men, as men are more likely to commit crime and are therefore more likely to be flagged incorrectly as riskier propositions.)
Any algorithm is only as good as the assumptions that you feed into it, and whether through deliberate malice or simple error in the case of soap dispensers, those assumptions can frequently be racist, sexist or classist.
But they are also better than what came before, not least because, when their assumptions and workings are publicly available we are better placed to work out why algorithms are producing racist, sexist or classist outcomes; and because even flawed algorithms tend to produce more fair results.
To take criminal justice as an example, study after study shows that humans in the criminal justice system produce judgements and make assessments that are fraught with racial or sexual prejudices. In a recent study, not only did 81 judges fail to unanimously agree on a single case out of 41 hypotheticals placed before them, the same judges ruled differently on identical cases when the only thing that changed was the suspect’s ethnicity or gender.
Even the algorithms that produce incorrect and prejudiced results more than half the time perform better than the humans they have replaced. To take the example of the Metropolitan Police’s use of facial recognition tech: just two out of the ten people it flagged were flagged correctly, an appalling result. But is quite literally 100 per cent more effective than the old powers of stop and search, where just one in ten stops ended in an arrest.
But, of course, it’s not good enough to declare that an algorithm is better than what it replaced. We can and should continue to refine prejudices out of the operation of algorithms, and there are two important aspects to that. The first, of course, is that the workings of algorithms need to be open-sourced and regularly tested. No algorithm that sets public policy should be kept away from public scrutiny or from academic observation. That bit is fairly easy, as the policy demand is simple and in parts of the public realm is already happening.
But the second and equally important part is that, when algorithms fail, we need to be able to discuss honestly and openly what has gone wrong. A soap dispenser that black people cannot use is racist: it doesn’t matter that the intentions of the people who made it were pure. What matters is the outcome. For the age of algorithm to produce fairer outcomes than the age of human intuition, as opposed to ones that are merely less unfair, people need to be able to discuss when algorithms fall short without being shouted down.