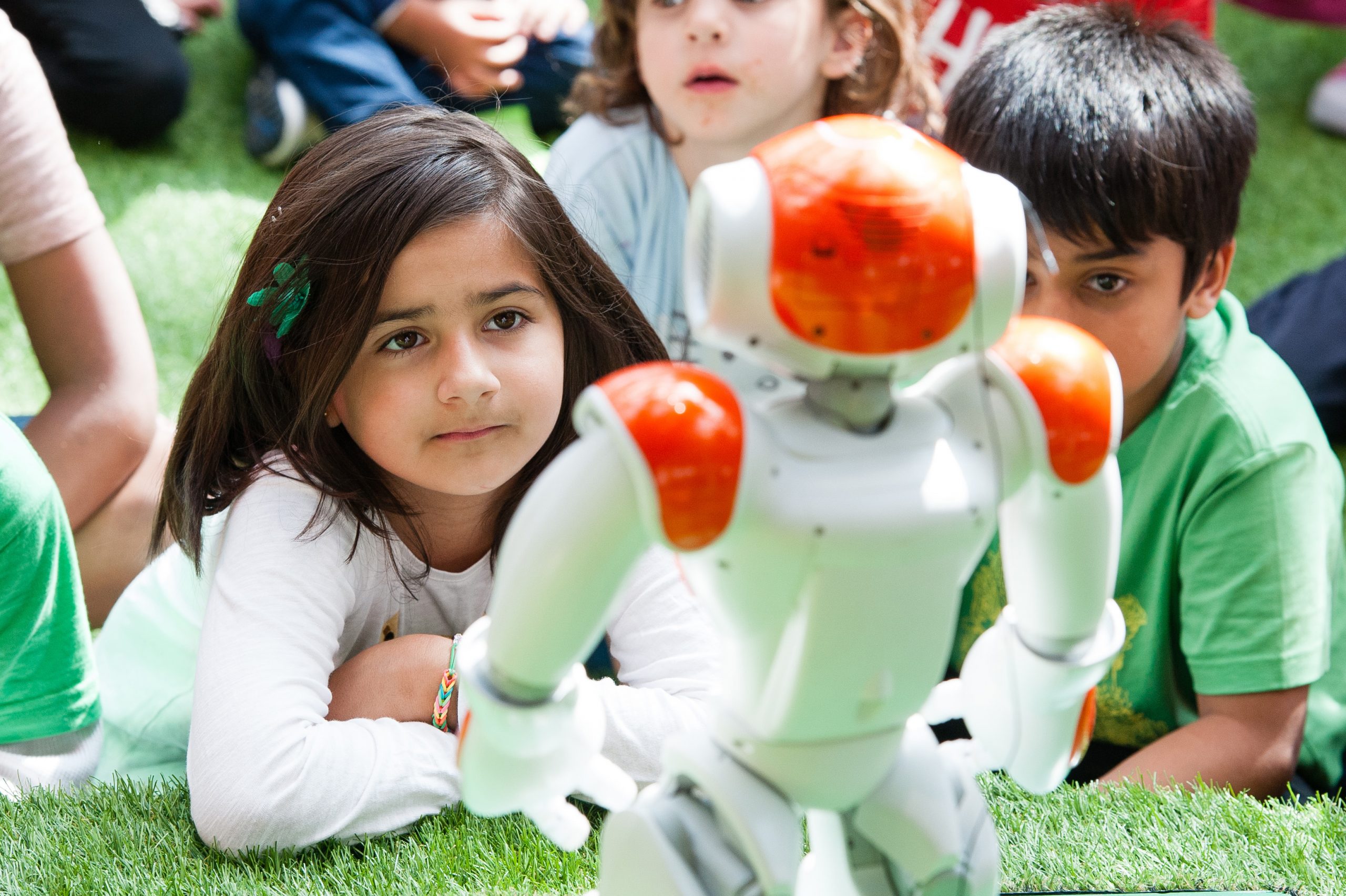
Throughout the world, artificial intelligence (AI) is increasingly being used to help make decisions about healthcare, loans, criminal sentences and job applications. For example, doctors throughout the US healthcare system use algorithms to make decisions about treatment.
However, a recent study shows that one such commonly used algorithm has significant racial bias. It is much more likely to determine that a white patient needs extra care than a black patient, even when the two patients have almost identical health issues. This algorithm and others like it have been used in the care of hundreds of million patients a year in the US. What is going on here?
Every natural and artificial recognition system uses statistical generalisations to operate efficiently. As a consequence, all natural and artificial recognitional systems have some biases. The human visual system, for instance, is riddled with them. Some of these biases are beneficial, some are harmful.
Our perceptual system, for example, assumes that light comes from above. This is a beneficial bias for us to have, since we live in environment in which light typically comes from above. Specifically, this bias makes the visual system more efficient by allowing us to process incoming information more efficiently. Occasionally, the assumption that light comes from above leads us to make mistakes, but for the most part all goes well.
While this light bias is unproblematic, visual biases are harmful if they generate perceptual states that reinforce injustices in our society or lead us to interact with others in harmful ways. Given how flawed humans are, it may not be surprising that our vision is biased, for in the end we are driven by fear, act on the basis of emotions, and are prone to misunderstandings.
It may, however, come as a surprise that AI systems are biased. After all, computer algorithms form the core of AI systems and these algorithms are grounded in mathematics and operate on data. So one might expect them to be objective and just. But they aren’t. There are two key ways in which algorithms may be biased: the data on which the algorithm is trained, and how the algorithm links features of the data on which it operates. We can call the first training-sample bias and the second feature-linking bias.
Let’s first look at training-sample bias. Google’s speech recognition algorithm is a good example. It performs better on male than on female voices. Why? Any complex algorithm is operationalised on data. Google’s speech recognition algorithm was trained disproportionately on the voices of men. As a consequence, the algorithm works best when decoding voices within the frequency of typical male voices.
There are many similar cases. A Nikon camera with built-in facial recognition software used to misrepresent Asian faces, asking the user: “Did someone blink?” In 2015, Google’s facial recognition algorithm identified two African Americans as gorillas. In both cases, the source of the problem can be traced back to the skewed data on which the algorithms were trained: their training data consisted primarily of caucasian faces.
What about the second source of algorithmic bias: feature-linking bias? AI systems operate on colossal and complex datasets. The datasets consist of pieces of information, which we can call features. Algorithms pick up on regularities and correlations between features, link these features, and then make predictions about the future. The correlations may be so complex as to be beyond human understanding even by the programmers who produced the initial algorithms. Yet they are not beyond algorithmic discovery. An example will help illustrate this.
In companies around the world, algorithms are used to filter promising job applications. These hiring algorithms can be biased in multiple ways. At Fox News, for example, an algorithm is used to determine a job application “promising” based on who was successful at Fox News in the past. Specifically, it focuses on two features: that the employee stayed for five years and was promoted twice during that time. The algorithm then filters for applicants that are similar to past employees who satisfy those two criteria.
Most Fox News employees who satisfy those two criteria in the past have been white men. Perhaps unsurprisingly, most of the job applications that the algorithm deems promising are the applications of white men. Ultimatley, this bias is due to the algorithm selecting and linking features in problematic ways, and the features these algorithms link can be as surprising as having played football in college.
There are feature-linking biases where the stakes are even higher. One is the Northpointe recidivism risk software COMPAS, which is used in courtrooms across the US. The software calculates a score predicting the likelihood of someone committing a crime in the future and judges use these recidivism risk scores to inform decisions about criminal sentencing.
As a ProPublica study revealed, the scores proved highly unreliable in predicting future crime: it was only marginally more reliable than a coin flip. Even more damning is that the algorithm generating the score is biased. It is much more likely to assume that African Americans are likely to reoffend. As a result, judges using this software are more likely to give African Americans harsh sentences.
What is the source of the bias? Northpointe’s scores are based on answers to a set of questions. While race is not asked about directly, the questions provide a host of information about poverty, joblessness and social marginalisation. The software then links features that are proxies for race, such as zip codes, in ways that leads to bias against African Americans.
Biased algorithms are a problem since they lead to biased outcomes. By repeating our past practices, algorithms not only automate the status quo and perpetrate bias and injustice, but they amplify the biases and injustices of our society.
To see this, let’s consider again the healthcare algorithms used throughout the US. The racial bias in these algorithms stem from the algorithm using past healthcare costs as a proxy for health. If a patient’s past healthcare costs were high, then the algorithm predicts that the patient should get extra care.
This would be unproblematic if there were a tight link between past healthcare costs and the need for extra medical care. But if past healthcare costs are more tightly linked to income, level of health insurance and access to medical care, then such an algorithm will amplify income and insurance-related injustices. Specifically, patients who had low healthcare costs in the past due to low income or inadequate health insurance will be less likely to get the extra medical care they need. In our imperfect world, biased algorithms generate harmful feedback loops and reinforce human prejudices.
What can be done to avoid biased algorithms? There are two key steps. We can expose them to balanced training samples, and we can check and correct how they link features. The difficult questions for AI systems are what it means for a computer algorithm to be fair. Should fairness involve making sure everyone has an equal probability of obtaining some benefit? Should it involve correcting for past injustices?
As the recent study on racial bias in healthcare treatment shows, there is an urgent need to fix algorithmic bias. With proper regulation we can design algorithms that ensure fair and equitable treatment for everyone. Currently there are no such regulations, but attempts are being made to require companies, agencies and hospitals to assess their use of automated decision systems.
In April 2019 US senators Cory Booker and Ron Wyden, along with representative Yvette D Clarke, introduced the Algorithmic Accountability Act. It requires companies to evaluate and fix biased computer algorithms that result in inaccurate, unfair, or discriminatory decisions. It is the first legislative effort to regulate AI systems across industries in the US. This is a good start.
Susanna Schellenberg is Professor of Philosophy and Cognitive Science at Rutgers University. She is the author of The Unity of Perception: Consciousness, Content, Evidence.
This article is part of the Agora series, a collaboration between the New Statesman and Aaron James Wendland, Professor of Philosophy at the Higher School of Economics. He tweets @ajwendland.