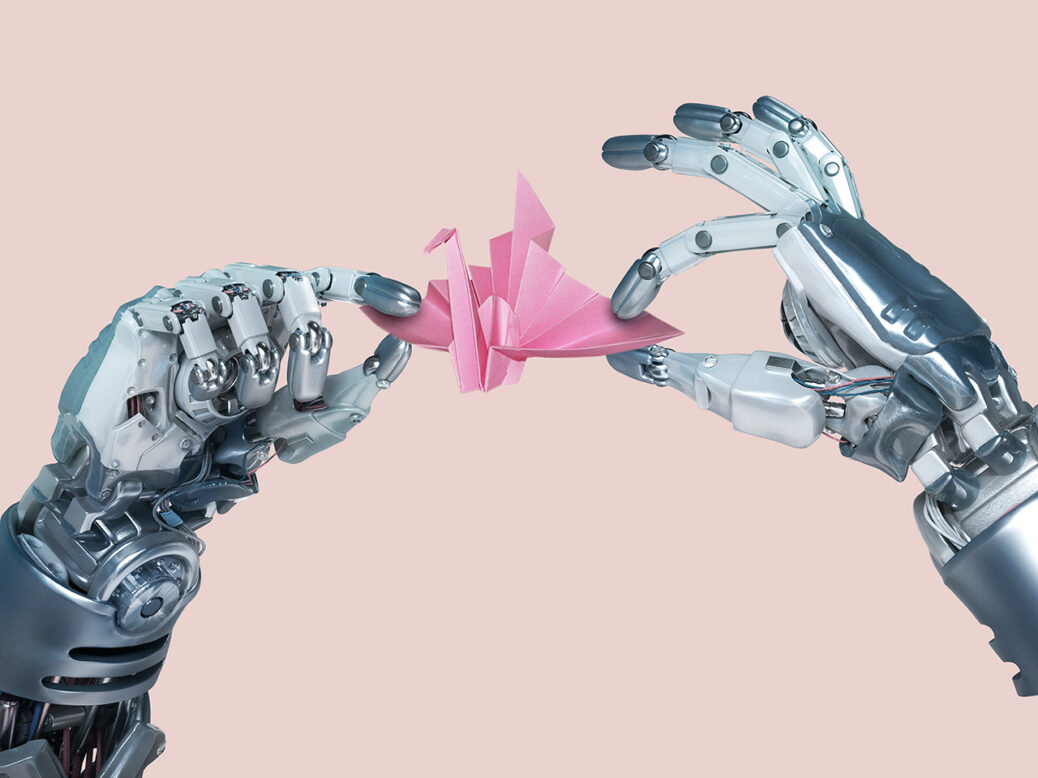
I’ve worked on artificial intelligence (AI) for 35 years. For most of that time it had a distressingly poor reputation. Academics tended to treat it with suspicion at best, outright hostility at worst, and the computing industry ignored it altogether. But about ten years ago something changed. Colleagues who would previously have spent their careers struggling to get research funds suddenly found themselves feted by the richest companies in the world and offered astonishing sums of money. Breathless stories started to appear in the press. What happened? Why had a field with a reputation akin to homeopathic medicine suddenly become the hottest area in tech?
What happened is that neural networks finally started to work.
Neural networks are like an animal brain: if you look at one under a microscope, you will see huge numbers of nerve cells, connected in enormous networks. These cells – neurons – are capable of recognising simple patterns in their network connections. When a neuron recognises a pattern, it communicates through electrochemical signals with its network neighbours. These neighbours are also looking for patterns in their connections, and when they see one, they too signal to their neighbours. And somehow, in ways we don’t quite understand, these enormous networks give rise to the miracle that is you. In the 1940s the American researchers Warren McCulloch and Walter Pitts were struck by the thought that the neural networks that we see in animal brains resemble electrical circuits – and this idea naturally led to the idea of building artificial neural networks. Thus was the field of neural networks born.
[See also: There is no chance the government will regulate AI]
Neural networks are an old idea, but the ingredients to build them only became available this century. To build one, you need lots of data – and we live in the age of big data. Every time you make a comment on social media or upload a picture of your dog having a bath, you are providing data for the neural networks of big tech companies. The second ingredient is computer power, and until recently power on the scale required for practical neural networks was too expensive to be feasible. Both of those ingredients – data and computer power – became abundant this century.
As the technology developed it became clear that size matters: the competence of a neural network scales with the size of the network, and the amount of data and computer power you throw at it. What followed was then perhaps predictable – a race to scale.
OpenAI’s ChatGPT, launched in November, and its recently-announced stablemate GPT-4, are the result of that rush to scale. GPT-4 is the fourth in a series of systems by OpenAI (despite the name, it is a for-profit company funded by Microsoft). OpenAI, founded by the Canadian AI researcher Ilya Sutskever in 2015, attracted ridicule when it claimed in 2019 that its GPT-2 program was too powerful to be made publicly available. Its successor GPT-3, announced in 2020, quickly silenced the cynics. GPT-3 represented a step change in capability over its predecessors – it could process and create language at the level of a graduate.
“Large language models,” like GPT-3 are actually absurdly simple. If I start a text message to my wife and type “Where are…”, my smartphone will suggest completions – the likeliest next words for my message. In this case, the completions might be “you” or “the kids”. My smartphone has seen all my text messages, and learned these are the commonest words after “Where are…”. GPT-3 does the same thing but on a much larger scale – the training data for GPT-3 included everything on the World Wide Web. The entirety of the web was ingested by GPT-3, requiring expensive supercomputers running for months to train its neural networks. That scale gave GPT-3 unprecedented capabilities. As one colleague put it, problems that AI researchers had been working on for decades were solved by GPT-3 almost as an afterthought. And remember, GPT-3 does nothing more than your smartphone when you start typing a message: predicting what comes next. It is ludicrous that such a simple idea can become something so powerful – particularly since we can’t even explain why it works.
[See also: Who’s really making money from HustleGPT?]
ChatGPT is a polished version of GPT-3. Upon release in late 2022 it immediately went viral – suddenly anybody in the world could interact with the most sophisticated AI in the world. And ChatGPT feels like the AI we were promised: you communicate with it like another human being, and it seems to understand. For all their limitations (they get things wrong, a lot), GPT-3 and ChatGPT started a wave of creative innovation. They will soon appear in your web browser, word processor, and email client. They will quietly help you with a thousand of the tedious tasks that make up much of your working life. Initially you’ll be impressed but, to the irritation of AI researchers, you’ll soon take it for granted. The recently announced GPT-4 marks another step forward. The key advance is that it is multi-modal: it works with text and images. This clearly signals where the technology is going – sound and video are next on the AI to-do list.
Systems like ChatGPT are not the end of the road for AI – not by a long way. For one thing, they are weirdly disembodied. They aren’t aware of our world, and don’t inhabit it in any real sense. They can’t tie a pair of shoelaces, cook an omelette, or tidy a room. Progress in robotic AI is slow, because dealing with the physical world is much, much harder than dealing with language. But nevertheless, after a decade of hype, we can all get our hands on AI: this is truly a watershed moment in technology.
[See also: Can AI automate human creativity?]