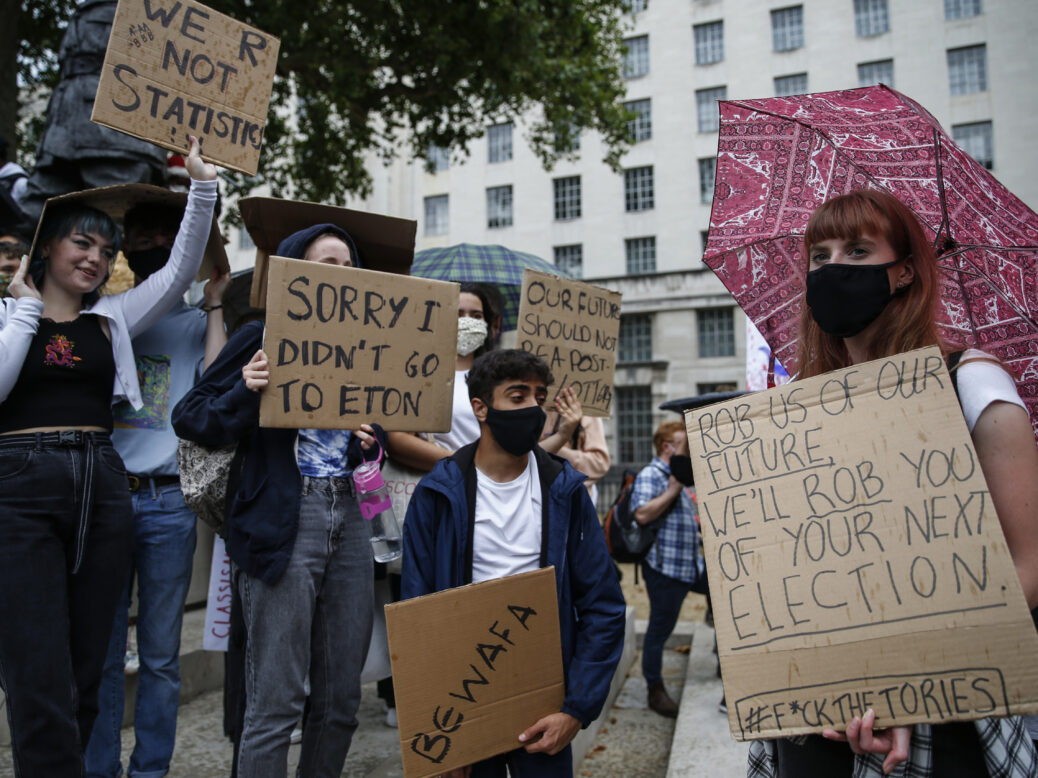
“All models are wrong,” statisticians like to remind us, following an aphorism coined (most likely) by British statistician George Box in 1976, “… but some are useful”. Of course, usefulness alone is often not all we want. You might worry about being assessed by a statistical model that makes judgements not strictly about you, today, as an individual, but rather about people like you, based on what they have done in the past. You might worry that although making abstract generalisations in this way might reveal some interesting patterns about people like you, it might ultimately fail to reflect what makes you unique, complicated, not fully predictable.
Statisticians will insist that with modelling there is no way around abstraction: we cannot build statistical models without abstracting from the real world in all its complexity, its messiness, and parcel it out into measurable morsels of information that can be expressed in binary terms. And in any case, if Box is right, not all forms of abstraction and generalisation are inherently objectionable in the first place: we should not reject a statistical model simply because it does not represent us and our reality fully, provided that the model is still able to tell us something interesting and illuminating about the social world. Sometimes, too much granular detail distracts us from the bigger picture, from what is really going on. Sometimes, the simplified version of the story is good enough.
Yesterday (13 August) was A-level results day in England, Wales and Northern Ireland. Owing to the cancellation of A-level and GCSE exams, the English regulator Ofqual’s statistical model determined students’ grades, based on their school’s recent exam history, as well as each student’s prior exam performance. This caused outrage: because Ofqual’s model seemed crude, because some students were cheated out of the opportunity to prove their teachers wrong by exceeding expectations, and because nearly 40 per cent of A-level results were downgraded.
Statistical models often seem rather opaque to us. Therefore, it’s unsurprising that many of us view them as something that risks increasing uncertainty. But actually, and counterintuitively, the opposite is the case. When we abstract and generalise, we artificially downplay uncertainty. We lose some leeway for giving people the benefit of the doubt. So, it is true that some models are useful – but some might not be.
[see also: A majority of UK voters think that teachers alone should set this year’s exam results]
Is Ofqual’s model useful? Well, that depends on what we are looking for. If all we want to do is even out the gap between schools which inflate grades and schools which do not, fine: statistical modelling, even in a rather reductive form, might be useful for this purpose. But on Ofqual’s model, the space for uncertainty, for giving the benefit of the doubt, does not shrink in the same way for everyone.
This is a major problem, albeit not the only one. A range of concerns have been voiced in recent weeks about Ofqual’s model: there is the (often unconscious) bias, for instance, that individual teachers might have against some of their students when evaluating their past performance, and predicting their future potential. But there is also the problem that different teachers will evaluate students differently, and in a way that is not easy to compare and standardise.
A more complex problem, described by the Royal Statistical Society (RSS) in an April 2020 letter to Ofqual, is that the grades of students who are neither very high nor very low achievers are “likely to be subject to more uncertainty (both in the sense of true uncertainty, and potentially systematic bias)” – a problem which could feasibly have been mitigated if Ofqual’s model had incorporated more sophisticated uncertainty estimates, as the RSS had urged. And Ofqual’s model is probably less accurate when determining results for subjects studied by a very small number of students in a given school, since less fine-grained data is available in those cases.
Yet another problem is that statistical models might “overfit” on past data, and thus fail to adequately reflect a changed status quo. This will particularly disadvantage students at formerly lower-achieving state schools that have rapidly improved of late, while students who have the privilege of attending a school with a long and consistent track record of overall high performance will be further privileged.
[see also: Top A-level grades soar at private schools as sixth form colleges lose out]
But most importantly, using statistical models for grade prediction, or for many other purposes – ranging from law enforcement and criminal justice, to models used in credit scoring and in allocating welfare benefits – raises a deeper political problem. Machine learning pioneer Ada Lovelace once observed that “the Analytical Engine weaves algebraic patterns just as the Jacquard loom weaves flowers and leaves”. That rings true today but for different, and less optimistic, reasons than the ones that Lovelace was probably thinking about.
Statistical models often pick up on large-scale historical patterns or racial, gender and class inequalities and replicate them as data patterns, while simultaneously endowing these patterns with an air of certainty, neutrality and objectivity. After all, numbers don’t have agendas, right? This is a dangerous illusion. How we choose to use statistical models, which questions we ask (and don’t ask) when we “simply look at the numbers” depends on political assumptions and priorities. If we do not carefully consider who merits the benefit of the doubt – who typically gets given more leeway to prove their potential due to their proximity to prestige, and who is more likely to be considered an inevitable product of a bad environment – then BAME students and working-class students will be further disadvantaged by the use of statistical inference methods.
[see also: For this year’s school leavers, a return to “normal” means dashed hopes and a loss of control]
We should treat this moment as an occasion to ask: when can the act of withholding judgement, and making space for uncertainty, ultimately promote social justice? Who is typically afforded, or denied, the space to impress and surprise? Who has the most to lose when the space of uncertainty shrinks?
Annette Zimmermann is a political philosopher at Princeton University working on the ethics of artificial intelligence and machine learning